How AI Could Help Solve the Enterprise Data Quality Dilemma
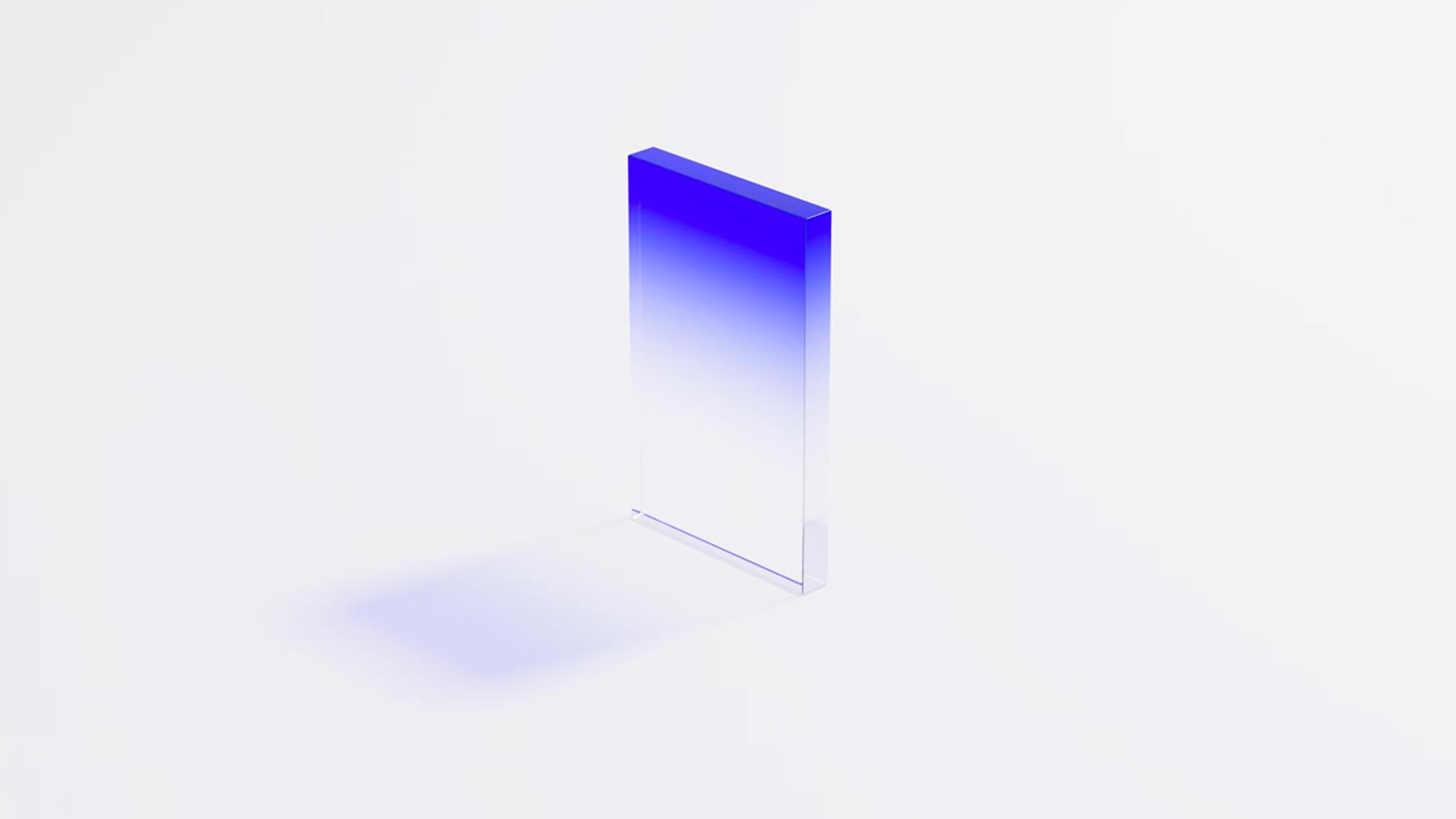
Today’s wave of AI technology is exposing the depths of enterprise data quality issues—but it could also be part of the solution.
AI’s potential elicits many reactions—terror, exuberance, skepticism—sometimes all at once. These days, my main feeling is hope. No, not about every impact AI may have, but specifically about one area that has languished despite all best efforts: data quality.
Some may scoff at such a suggestion. Data quality—which is an outcome, not a tool—remains an elusive goal for many enterprises, and the current wave of AI technology is EXACERBATING the problem.
In fact, some will rightly note that this is the third wave of data quality’s demise. The first was the transition to the cloud, which took data from contained basement servers to the modular, accessible, connected data warehouse. The second was the rise of the modern data stack, which siloed data across tools. Data observability, data storage, data processing, data search—each new solution introduced more opportunities for data quality to be degraded.
Once again, things are looking bleak (but bear with me, there’s a light at the end of this article). Enterprises are eager to leverage AI internally and in customer-facing products, but since data quality directly impacts the accuracy of AI models, their success with AI is dependent on the data they have. Teams are finding they lack high quality data across their vast and siloed stores. Many are resorting to zero-shot learning, in which models learn to classify data it has not seen before.
Not to mention, AI has driven increased scrutiny on data (quality, accuracy, provenance, etc.) because engineers do not entirely understand how data sets impact AI models. As leaders leverage nondeterministic algorithms to direct business outcomes, the correctness and explainability of those models and the data that went into training them will be audited. Incomplete data can lead to inaccurate and biased outcomes, a point outlined in a 2019 EU agency paper. The provenance of model behavior comes down to data quality, so it will need to be governed differently than ever before.
Here is where hope factors in: With greater responsibility come greater opportunities and resources to advance.
The paradigms that have been introduced over the years to combat the data quality dilemma won’t suffice—and we believe enterprises will finally invest in real efforts to improve them.
Think about it: Solutions like data meshes that ensure data lineage and access control (well done, JP Morgan), or observability tools that report on data’s health and performance, or anomaly detection platforms that point out when data is not in acceptable range—these all try to solve data quality by reducing complexity. But as AI use permeates organizations, increased complexity is inevitable. A modern data quality solution will need to start at data production—automatically and intelligently cleaning data. Only then could it embed itself across a stack to ensure data quality is tracked, verifiable, and leveraged appropriately.
What could an AI-led solution look like?
The acceleration of AI adoption requires something new—and that new solution could include, perhaps unsurprisingly, AI itself.
Until recent years, research around improving data quality relied primarily on statistical models. For example, one 2018 paper out of Advances in Intelligent Systems and Computing outlined how combining deep learning and statistical models improved data quality; however, they merely used deep learning for prediction, and relied wholly on statistical models for outlier detection.
There is an opportunity for AI to be leveraged more directly to replace rules-based or statistical models to identify outliers and clean data. A more recent real-world example published in 2021 demonstrated how AI—when trained on valid and non-valid data points—could evaluate the quality of real-time drilling data for an oil and gas company. The main disadvantage here is that manual data preparation would be required to first train that model—specifically labeling accurate vs. inaccurate data—but theoretically this could work across use cases. It’s also important to note that the 78% accuracy their artificial neural network (ANN) achieved is not good enough to completely eliminate humans in the loop, and that for any latency-impacted use cases, this methodology would not be an option as the ANN was uniquely slow (slower, but more accurate, than support vector machine or decision tree models).
Some existing data quality software providers are incorporating AI into their products. Collibra, which started as a data catalog solution, has a newer Data Quality and Observability product that relies on AI-generated “AdaptiveRules.” This product determines trends to code data quality rules automatically. Monte Carlo publicly discusses how they leverage ML to automatically test data and surface recommendations. The data labeling providers (Snorkel, Encord, etc.) have also leveraged programmatic labeling effectively, and ML-methods for data cleaning have been oft articulated (see Data Cleaning, 2019).
How good is good enough?
Training and running AI models for data quality improvements—like any use case—is neither cheap nor easy. So the question of enterprises is this: what data should I invest in, and what level of data quality is good enough? Data quality management will vary in its precision and depth based on the value of business data. And for specific use cases, enterprises will have to decide what level of accuracy (a reflection of data quality) is good enough.
We recommend a practical approach. Consider the risk of being wrong vs. the cost of getting it right:

High risk, low cost: With financial reporting, even small errors could result in fraud charges. Yet getting the data underlying the P&L in good shape is one of the most well worn data quality patterns. Detailed, but not difficult, with many products available to make high quality automation feasible.
High risk, high cost: Enterprises are being told that the more unique and proprietary their data, the more extraordinary their AI products can be. But the more unique the data, the more complex the process of wrangling it and making it usable. Think of Schlumberger, the oil and gas innovator. The data they have access to, like telemetry from bespoke gear, is odd and difficult to interpret. They have built their own embedded AI data solution to ensure their customers can access data in the right context and make better decisions. The consequences of using that data incorrectly are real, and the cost to ensure data quality and its ease of consumption is high.
Low risk, high cost: With endless customer behavior data, personalizing products and ads has significant benefits: lower cart abandonment, less choice paralysis, more customer satisfaction. Plus, poor recommendations for marginal customers are of little concern. However, Facebook's data system—used predominantly for personalisation—is one of the largest and most expensive in the world. Personalization at scale is only a well worn path for the most advanced tech companies, and most enterprises would need to make a massive investment to achieve similar results.
Low risk, low cost: Most consumers have now engaged with support bots powered by AI summarization and AI-driven search. There is more than enough text data available to train these models without a heavy data quality lift. The end results are mediocre, at best, but if a customer cannot get what they need in a chat, they can always call and ask to speak to a human (for now).
Until data quality solutions are consistently efficacious and cost effective, enterprises will need to prioritize which data is most important. Business critical data must be the highest quality. Over time, the cost to achieve high data quality will go down, and as new solutions become available—perhaps AI-led themselves—companies will have the flexibility to explore the value of the terabytes of data currently unused and undiscovered.
Today’s wave of AI technology is exposing the depths of enterprise data quality issues—but it could also be part of the solution.
AI’s potential elicits many reactions—terror, exuberance, skepticism—sometimes all at once. These days, my main feeling is hope. No, not about every impact AI may have, but specifically about one area that has languished despite all best efforts: data quality.
Some may scoff at such a suggestion. Data quality—which is an outcome, not a tool—remains an elusive goal for many enterprises, and the current wave of AI technology is EXACERBATING the problem.
In fact, some will rightly note that this is the third wave of data quality’s demise. The first was the transition to the cloud, which took data from contained basement servers to the modular, accessible, connected data warehouse. The second was the rise of the modern data stack, which siloed data across tools. Data observability, data storage, data processing, data search—each new solution introduced more opportunities for data quality to be degraded.
Once again, things are looking bleak (but bear with me, there’s a light at the end of this article). Enterprises are eager to leverage AI internally and in customer-facing products, but since data quality directly impacts the accuracy of AI models, their success with AI is dependent on the data they have. Teams are finding they lack high quality data across their vast and siloed stores. Many are resorting to zero-shot learning, in which models learn to classify data it has not seen before.
Not to mention, AI has driven increased scrutiny on data (quality, accuracy, provenance, etc.) because engineers do not entirely understand how data sets impact AI models. As leaders leverage nondeterministic algorithms to direct business outcomes, the correctness and explainability of those models and the data that went into training them will be audited. Incomplete data can lead to inaccurate and biased outcomes, a point outlined in a 2019 EU agency paper. The provenance of model behavior comes down to data quality, so it will need to be governed differently than ever before.
Here is where hope factors in: With greater responsibility come greater opportunities and resources to advance.
The paradigms that have been introduced over the years to combat the data quality dilemma won’t suffice—and we believe enterprises will finally invest in real efforts to improve them.
Think about it: Solutions like data meshes that ensure data lineage and access control (well done, JP Morgan), or observability tools that report on data’s health and performance, or anomaly detection platforms that point out when data is not in acceptable range—these all try to solve data quality by reducing complexity. But as AI use permeates organizations, increased complexity is inevitable. A modern data quality solution will need to start at data production—automatically and intelligently cleaning data. Only then could it embed itself across a stack to ensure data quality is tracked, verifiable, and leveraged appropriately.
What could an AI-led solution look like?
The acceleration of AI adoption requires something new—and that new solution could include, perhaps unsurprisingly, AI itself.
Until recent years, research around improving data quality relied primarily on statistical models. For example, one 2018 paper out of Advances in Intelligent Systems and Computing outlined how combining deep learning and statistical models improved data quality; however, they merely used deep learning for prediction, and relied wholly on statistical models for outlier detection.
There is an opportunity for AI to be leveraged more directly to replace rules-based or statistical models to identify outliers and clean data. A more recent real-world example published in 2021 demonstrated how AI—when trained on valid and non-valid data points—could evaluate the quality of real-time drilling data for an oil and gas company. The main disadvantage here is that manual data preparation would be required to first train that model—specifically labeling accurate vs. inaccurate data—but theoretically this could work across use cases. It’s also important to note that the 78% accuracy their artificial neural network (ANN) achieved is not good enough to completely eliminate humans in the loop, and that for any latency-impacted use cases, this methodology would not be an option as the ANN was uniquely slow (slower, but more accurate, than support vector machine or decision tree models).
Some existing data quality software providers are incorporating AI into their products. Collibra, which started as a data catalog solution, has a newer Data Quality and Observability product that relies on AI-generated “AdaptiveRules.” This product determines trends to code data quality rules automatically. Monte Carlo publicly discusses how they leverage ML to automatically test data and surface recommendations. The data labeling providers (Snorkel, Encord, etc.) have also leveraged programmatic labeling effectively, and ML-methods for data cleaning have been oft articulated (see Data Cleaning, 2019).
How good is good enough?
Training and running AI models for data quality improvements—like any use case—is neither cheap nor easy. So the question of enterprises is this: what data should I invest in, and what level of data quality is good enough? Data quality management will vary in its precision and depth based on the value of business data. And for specific use cases, enterprises will have to decide what level of accuracy (a reflection of data quality) is good enough.
We recommend a practical approach. Consider the risk of being wrong vs. the cost of getting it right:

High risk, low cost: With financial reporting, even small errors could result in fraud charges. Yet getting the data underlying the P&L in good shape is one of the most well worn data quality patterns. Detailed, but not difficult, with many products available to make high quality automation feasible.
High risk, high cost: Enterprises are being told that the more unique and proprietary their data, the more extraordinary their AI products can be. But the more unique the data, the more complex the process of wrangling it and making it usable. Think of Schlumberger, the oil and gas innovator. The data they have access to, like telemetry from bespoke gear, is odd and difficult to interpret. They have built their own embedded AI data solution to ensure their customers can access data in the right context and make better decisions. The consequences of using that data incorrectly are real, and the cost to ensure data quality and its ease of consumption is high.
Low risk, high cost: With endless customer behavior data, personalizing products and ads has significant benefits: lower cart abandonment, less choice paralysis, more customer satisfaction. Plus, poor recommendations for marginal customers are of little concern. However, Facebook's data system—used predominantly for personalisation—is one of the largest and most expensive in the world. Personalization at scale is only a well worn path for the most advanced tech companies, and most enterprises would need to make a massive investment to achieve similar results.
Low risk, low cost: Most consumers have now engaged with support bots powered by AI summarization and AI-driven search. There is more than enough text data available to train these models without a heavy data quality lift. The end results are mediocre, at best, but if a customer cannot get what they need in a chat, they can always call and ask to speak to a human (for now).
Until data quality solutions are consistently efficacious and cost effective, enterprises will need to prioritize which data is most important. Business critical data must be the highest quality. Over time, the cost to achieve high data quality will go down, and as new solutions become available—perhaps AI-led themselves—companies will have the flexibility to explore the value of the terabytes of data currently unused and undiscovered.